Graph Sampling with Determinantal Point Processes
- Imprimer
- Partager
- Partager sur Facebook
- Share on X
- Partager sur LinkedIn
Projet exploratoire
L'une des premières étapes nécessaires à l'exploration de données consiste souvent à réduire la taille des données d'intérêt afin d'exécuter les algorithmes d'exploration dans un délai raisonnable. À cette fin, les données sont généralement échantillonnées. Qu'il s'agisse d'un échantillonnage périodique ou à l'aide de combinaisons linéaires aléatoires, comme dans la détection compressée, la connaissance de la structure particulière des données permet de concevoir des schémas d'échantillonnage appropriés, efficaces (encodant efficacement l'information) et robustes au bruit. Dans le contexte actuel où les données sont de plus en plus mesurées sur des réseaux, il est nécessaire de fournir des schémas d'échantillonnage efficaces pour les signaux définis sur de telles structures irrégulières.
Résumé
Les graphes sont un outil essentiel de modélisation des données structurées en réseau. Selon l'application, les nœuds d'un graphe peuvent représenter des individus dans des réseaux sociaux, des régions cérébrales dans des réseaux neuronaux… en bref, tout système constitué de sous-systèmes interconnectés.
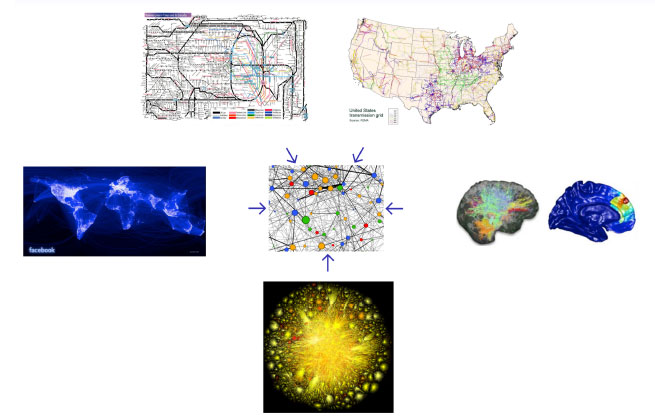
Tous ces éléments peuvent être modélisés à l'aide de graphes, objets mathématiques constitués de nœuds reliés par des liens, dont un exemple est illustré au centre.
Les données d'un graphe, appelées signaux de graphe, telles que les loisirs individuels ou le flux sanguin des régions cérébrales, peuvent être représentées par un scalaire par nœud. L'échantillonnage de graphe consiste à mesurer un signal de graphe lisse a priori sur un ensemble réduit de nœuds soigneusement choisis pour permettre une reconstruction stable. Dans des travaux antérieurs, nous avons démontré que ce problème est étroitement lié aux leverage scores, un concept essentiel dans les efforts de réduction de dimension tels que l'approximation de bas rang de grandes matrices. Afin d'améliorer les théorèmes existants en termes de nombre de nœuds nécessaires à l'échantillonnage, de robustesse au bruit de la récupération et/ou de complexité des algorithmes d'échantillonnage, nous proposons d'examiner de près les processus déterminants. En nous appuyant sur des résultats récents reliant les processus déterminants et les forêts couvrantes à racines aléatoires sur les graphes, nous étudierons comment des marches aléatoires particulières sur les graphes peuvent révéler des ensembles d'échantillonnage optimaux. Les résultats de cette étude pourraient avoir un impact important non seulement sur le traitement du signal des graphes, mais plus généralement sur l'apprentissage automatique et l'exploration de données, où la réduction de la dimension des données est souvent une étape nécessaire.
Résultats attendus
Nous nous appuyons sur des résultats récents sur les processus déterminants afin d'améliorer les algorithmes d'échantillonnage de graphes les plus récents. Les résultats de cette recherche pourraient avoir un impact dans le domaine des GSP, et plus généralement dans tous les domaines où la réduction de dimension via les scores de levier est importante.
Porteur
Nicolas Tremblay (Gipsa-lab)
Références
Illustrations
[1] Une illustration du réseau social Facebook ; intégrée sur la carte du monde.
[2] (À gauche) représentation d'un réseau neuronal, (à droite) représentation d'une ondelette sur le graphe modélisant ce réseau.
[3] Une carte de l'Internet, projet OPTE.
[4] Une carte non officielle du réseau de trains de banlieue et de métro de Tokyo.
[5] Le réseau électrique des États-Unis.
Publications
[1] A. Agaskar and Y. Lu. A Spectral Graph Uncertainty Principle. Information Theory, IEEE Transactions on, 59(7):4338{4356, July 2013.
[2] A. Anis, A. Gadde, and A. Ortega. Towards a sampling theorem for signals on arbitrary graphs. In Acoustics, Speech and Signal Processing (ICASSP), 2014 IEEE International Conference on, pages 3864{3868, May 2014.
[3] A. Anis, A. Gadde, and A. Ortega. Efficient Sampling Set Selection for Bandlimited Graph Signals Using Graph Spectral Proxies. IEEE Transactions on Signal Processing, 64(14):3775{3789, July 2016.
[4] L. Avena and A. Gaudilliere. On some random forests with determinantal roots. arXiv preprint arXiv:1310.1723, 2013.
[5] F. A. Azevedo, L. R. Carvalho, L. T. Grinberg, J. M. Farfel, R. E. Ferretti, R. E. Leite, R. Lent, S. Herculano-Houzel, and others. Equal numbers of neuronal and nonneuronal cells make the human brain an isometrically scaled-up primate brain. Journal of Comparative Neurology, 513(5):532{541, 2009.
[6] J. J. Benedetto. Irregular sampling and frames. wavelets: A Tutorial in Theory and Applications, 2:445{507, 1992.
[7] E. J. Candes and others. Compressive sampling. In Proceedings of the international congress of mathematicians, volume 3, pages 1433{1452. Madrid, Spain, 2006.
[8] S. Chen, A. Sandryhaila, and J. Kovacevic. Sampling theory for graph signals. In Acoustics, Speech and Signal Processing (ICASSP), 2015 IEEE International Conference on, pages 3392{3396, Apr. 2015.
[9] S. Chen, R. Varma, A. Sandryhaila, and J. Kovacevic. Discrete Signal Processing on Graphs: Sampling Theory. CoRR, abs/1503.05432, 2015.
[10] F. Chung. Spectral graph theory. Number 92. Amer Mathematical Society, 1997.
[11] R. R. Coifman and M. Maggioni. Diffusion wavelets. Applied and Computational Harmonic Analysis, 21(1):53{94, July 2006.
[12] D. A. Drachman. Do we have brain to spare? Neurology, 64(12):2004{2005, 2005.
[13] P. Drineas, M. Magdon-Ismail, M. W. Mahoney, and D. P. Woodruff. Fast approximation of matrix coherence and statistical leverage. The Journal of Machine Learning Research, 13(1):3475{3506, 2012.
[14] J. A. Dunne, R. J. Williams, and N. D. Martinez. Network structure and robustness of marine food webs. Marine Ecology Progress Series, 273:291{302, 2004.
[15] P. Gai and S. Kapadia. Contagion in financial networks. Proceedings of the Royal Society of London A: Mathematical, Physical and Engineering Sciences, 2010.
[16] B. Girault, P. Goncalves, and E. Fleury. Translation on Graphs: An Isometric Shift Operator. Signal Processing Letters, IEEE, 22(12):2416{2420, Dec. 2015.
[17] K. Grochenig. Reconstruction algorithms in irregular sampling. Mathematics of Computation, 59(199):181{194, 1992.
[18] D. Hammond, P. Vandergheynst, and R. Gribonval. Wavelets on graphs via spectral graph theory. Applied and Computational Harmonic Analysis, 30(2):129{150, 2011.
[19] P. Holme, B. J. Kim, C. N. Yoon, and S. K. Han. Attack vulnerability of complex networks. Physical Review E, 65(5):056109, 2002.
[20] C. J. Honey, J.-P. Thivierge, and O. Sporns. Can structure predict function in the human brain? Neuroimage, 52(3):766{776, 2010.
[21] H. Jeong, S. P. Mason, A.-L. Barabasi, and Z. N. Oltvai. Lethality and centrality in protein networks. Nature, 411(6833):41{42, 2001.
[22] A. Kulesza and B. Taskar. Determinantal Point Processes for Machine Learning. Foundations and TrendsR
in Machine Learning, 5(2{3):123{286, 2012.
[23] M. W. Mahoney. Randomized Algorithms for Matrices and Data. Foundations and TrendsR
in Machine Learning, 3(2):123{224, 2011.
[24] J. D. McEwen and Y. Wiaux. A Novel Sampling Theorem on the Sphere. IEEE Trans. Signal Process., 59(12), 2011.
[25] S. Narang and A. Ortega. Perfect Reconstruction Two-Channel Wavelet Filter Banks for Graph Structured Data. Signal Processing, IEEE Transactions on, 60(6):2786{2799, June 2012.
[26] S. Narang and A. Ortega. Compact Support Biorthogonal Wavelet Filterbanks for Arbitrary Undirected Graphs. Signal Processing, IEEE Transactions on, 61(19):4673{4685, Oct. 2013.
[27] B. Pasdeloup, R. Alami, V. Gripon, and M. Rabbat. Toward an uncertainty principle for weighted graphs. In 2015 23rd European Signal Processing Conference (EUSIPCO), pages 1496{1500, Aug. 2015.
[28] N. Perraudin and P. Vandergheynst. Stationary signal processing on graphs. arXiv preprint arXiv:1601.02522, 2016.
[29] I. Pesenson. Sampling in Paley-Wiener spaces on combinatorial graphs. Transactions of the American Mathematical Society, 360(10):5603{5627, 2008.
[30] I. Z. Pesenson and M. Z. Pesenson. Sampling, Filtering and Sparse Approximations on Combinatorial Graphs. J. Fourier Anal. Appl., 16(6):921{942, 2010.
[31] G. Puy, N. Tremblay, R. Gribonval, and P. Vandergheynst. Random sampling of bandlimited signals on graphs. Applied and Computational Harmonic Analysis, pages {, 2016.
[32] A. Sakiyama and Y. Tanaka. Oversampled Graph Laplacian Matrix for Graph Filter Banks. Signal Processing, IEEE Transactions on, 62(24):6425{6437, Dec. 2014.
[33] A. Sandryhaila and J. Moura. Discrete signal processing on graphs: Graph fourier transform. In Acoustics, Speech and Signal Processing (ICASSP), 2013 IEEE International Conference on, pages 6167{6170, May 2013.
[34] A. Sandryhaila and J. Moura. Big Data Analysis with Signal Processing on Graphs: Representation and processing of massive data sets with irregular structure. Signal Processing Magazine, IEEE, 31(5):80{90, Sept. 2014.
[35] C. Shannon. Communication in the Presence of Noise. Proceedings of the IRE, 37(1):10{21, Jan. 1949.
[36] D. Shuman, S. Narang, P. Frossard, A. Ortega, and P. Vandergheynst. The emerging field of signal processing on graphs: Extending highdimensional data analysis to networks and other irregular domains. Signal Processing Magazine, IEEE, 30(3):83{98, May 2013.
[37] D. I. Shuman, B. Ricaud, and P. Vandergheynst. Vertex-frequency analysis on graphs. Applied and Computational Harmonic Analysis, 40(2):260{291, Mar. 2016. in press.
[38] N. Tremblay and P. Borgnat. Subgraph-Based Filterbanks for Graph Signals. IEEE Transactions on Signal Processing, 64(15):3827{3840, Aug. 2016.
[39] N. Tremblay, G. Puy, R. Gribonval, and P. Vandergheynst. Compressive spectral clustering. In Proceedings of the 33 rd International Conference on Machine Learning (ICML), volume 48, pages 1002{1011. JMLR: W&CP, 2016.
[40] C. Wilks and P. Meara. Untangling word webs: graph theory and the notion of density in second language word association networks. Second Language Research, 18(4):303{324, 2002.
- Imprimer
- Partager
- Partager sur Facebook
- Share on X
- Partager sur LinkedIn